For Aspiring Data Scientists, This Is All About Your Dream Jobs
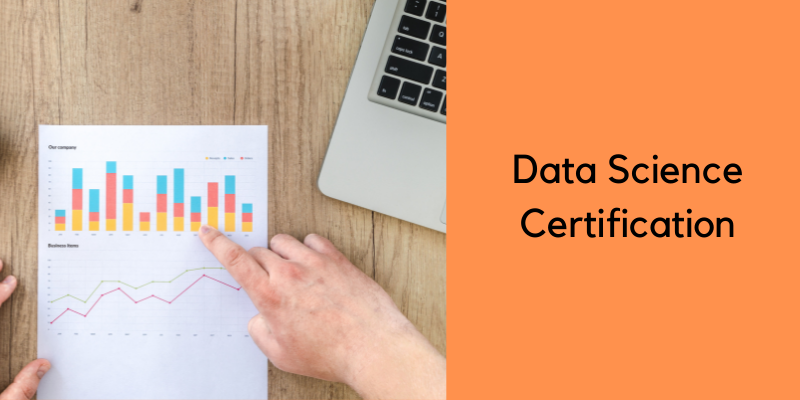
When the planet moved into the era of big data, so did the need for storage. Until 2010, it was the biggest threat and source of concern for the business sectors. The primary emphasis was on developing a platform and data storage strategies. Now that Hadoop and other architectures have effectively solved the storage challenge, the emphasis has turned to data processing. The secret sauce here is data science. Data Science will make all of the theories that you see in Hollywood sci-fi movies a reality. Artificial Intelligence’s future is Data Science. As a result, it’s critical to comprehend what Data Science is and how it can benefit the business.
Data Science is a set of software, algorithms, and machine learning concepts aimed at uncovering secret patterns in large amounts of data. The Data Analyst typically discusses what’s going on by going through the data’s processing history. On the other hand, a Data Scientist not only does exploratory research to uncover insights but also employs sophisticated machine learning algorithms to predict the occurrence of a certain event in the future. A Data Scientist can examine the data from various perspectives, including those that were previously unknown. Thus, predictive causal analytics, prescriptive analytics, and data science are mainly used to make conclusions and forecasts.
Data scientists are experts in specific research fields and use their knowledge to solve complicated data problems. They deal with various topics such as geometry, statistics, and computer science (though they may not be an expert in all these fields). They make extensive use of cutting-edge technology to identify alternatives and reach critical decisions about an organisation’s growth and progress. Data Scientists view data in a far more usable format than the raw data they have access to in both organised and unstructured formats.
A Data Science Life cycle:
Discovery:It’s critical to consider the different criteria, expectations, goals, and budget requirements before starting the project. You must be able to ask the appropriate questions. This is where you determine whether you have the necessary capital in terms of manpower, technologies, time, and data to fund the project. You must also frame the market dilemma and devise initial theories (IH) to evaluate during this process.
Data preparation:You’ll need an analytical sandbox for this process, where you can run analytics for the length of the project. Before modelling, you must explore, preprocess, and state info. To bring data into the sandbox, you’ll use ETLT (extract, convert, load, and transform). Have a peek at the Statistical Analysis flow in the diagram below.
Model planning:Here, you’ll figure out how to draw relationships between variables using various methods and techniques. These relationships will serve as the foundation for the algorithms you’ll use in the next step. You’ll use different mathematical formulas and simulation software to implement Exploratory Data Analytics (EDA).
Model building:You will build datasets for training and research in this process. Consider if your current resources would suffice for running the models or whether a more stable environment is needed (like fast and parallel processing). To construct the model, you will examine different learning strategies such as grouping, correlation, and clustering.
Operationalise: Final reports, briefings, code, and technical documentation are delivered during this process. Additionally, a pilot experiment can be applied in a real-time manufacturing environment on occasion. This will give you a good view of the results and other associated restrictions on a small scale before deploying it on a larger scale.
Communicate results: It’s now time to assess whether you successfully achieved the target you set for yourself in the first round. So, in the final process, you’ll define all of the main conclusions, explain them to the stakeholders, and assess whether the project’s outcomes were a success or a loss based on the parameters established in Phase 1.
Why go through a Data Science Certification Course?
We live in an environment of cutting-edge technology, which has resulted in a data explosion. As a result, quicker and improved inventions and intelligent goods have become a reality. In the past decade, the amount of data available and the desire to harness it has increased dramatically, allowing companies to focus their operations on data, make smarter choices and create new job positions.
A Data Science Certification Course will teach you everything you need to know about data science, including what it is, what career options are available in the field, the differences between a data scientist, data analyst, and data engineer jobs, and the subsequent technologies associated with it. It would be your entry ticket into the industry or a prestigious degree course in Data sciences or machine learning courses at leading colleges. These are very impressive to write in your resume as well.
The data science courses encompass a wide range of skills and subjects from logistic regression to decision trees, SVM, and linear regression. So, apply for data science at platforms like CareerEx and along with a machine learning course to pick up some crucial skills to help you become a data science pro!